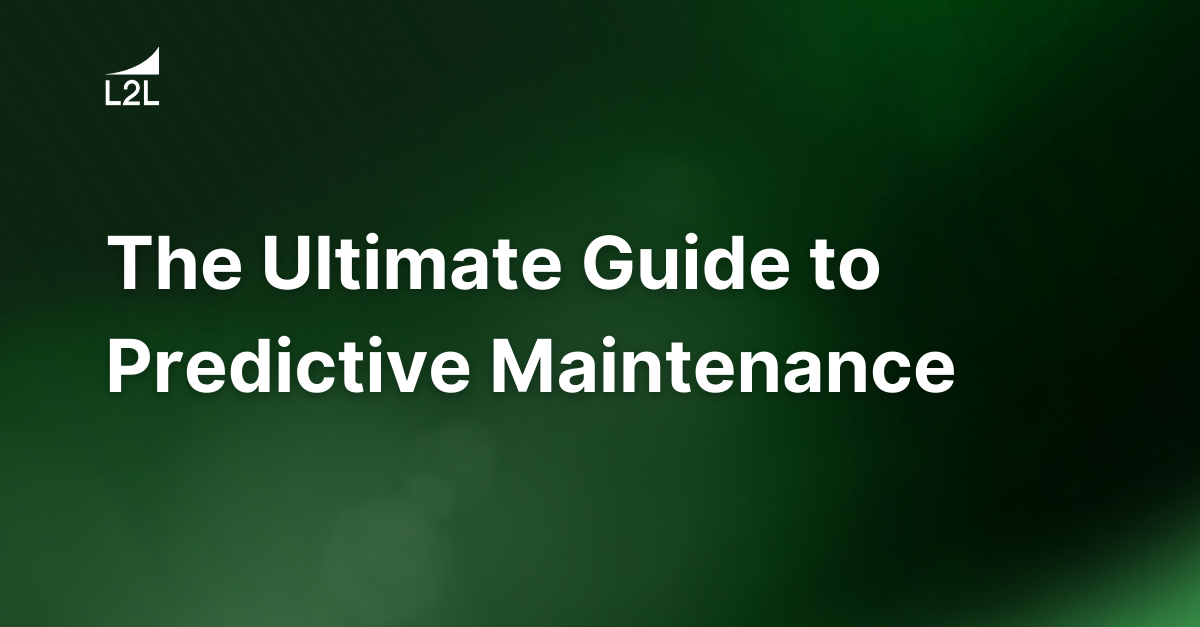
Maintaining equipment is a critical part of keeping any manufacturing operation running smoothly. But traditional approaches to maintenance, like routine inspections or reactive repairs, can lead to inefficiencies, unplanned downtime, and high costs. That’s what predictive maintenance is trying to prevent.
Predictive maintenance uses real-time data and AI to anticipate equipment failures before they happen. By using sensors, data analytics, and machine learning, companies can predict when a machine is likely to fail — and take action to prevent that failure.
In this article, we’ll break down how predictive maintenance works, the key technologies involved, and why so many companies are moving toward this maintenance strategy.
What is predictive maintenance?
Predictive maintenance (PdM) is an advanced approach to maintaining equipment, where condition monitoring data and predictive analytics are used to forecast when equipment is likely to fail. This allows companies to perform maintenance at the optimal time — just before a breakdown would occur — minimizing production disruptions and maximizing asset lifespan.
Unlike preventive maintenance, which follows a set schedule for repairs or replacements regardless of the equipment’s actual condition, predictive maintenance uses real-time data to base maintenance decisions on the actual performance and health of each asset. This means maintenance only happens when it’s necessary.
Why do companies employ predictive maintenance?
Predictive maintenance has gained traction in industries like manufacturing because it offers a range of significant benefits that traditional maintenance strategies simply can’t match.
U.S. PdM market growth projection. Source: GVR
Here are a few key advantages of PdM that are responsible for this increased adoption:
-
Optimized asset performance and productivity: By predicting equipment failures, companies can keep their machines running at peak performance with minimal disruptions. Assets are only taken offline when absolutely necessary, leading to improved productivity.
-
Extended asset lifespan: Performing maintenance based on actual equipment conditions helps reduce unnecessary wear and tear. This extends the life of critical machinery, delaying costly replacements.
-
Minimized planned and unplanned downtime: By performing maintenance at the right time, companies can avoid expensive breakdowns and optimize their production schedules and management.
-
Cost savings from optimized spare parts management: Since predictive maintenance allows companies to know exactly when parts will need replacing, it helps optimize spare parts inventory. This means less money is tied up in unnecessary stock and fewer delays waiting for parts when maintenance is required.
While the benefits are clear, implementing a predictive maintenance program isn’t without challenges. It often comes with high startup costs, as the technology and infrastructure needed to gather and analyze data can be expensive. It also requires large amounts of data to deliver accurate predictions, which may not always be readily available. Finally, companies must invest in workforce training and upskilling to ensure their teams can operate the technology and interpret the results effectively.
That said, considering market projections and our own experience, the benefits you get are worth the trouble.
Technology involved in running predictive maintenance
Predictive maintenance relies heavily on modern technology to gather, analyze, and act on real-time data from machines. This tech ecosystem combines various tools, sensors, and software to monitor the condition of assets and predict when maintenance is needed.
We will use the following sections to explain the core components of a predictive maintenance system and showcase how predictive maintenance works.
Condition monitoring and IoT to pick up real-time data
The backbone of predictive maintenance lies in condition monitoring, where data is collected from machines in real time using various sensors. These sensors, connected through the Internet of Things (IoT), constantly track the health of equipment and detect changes in performance.
This data helps identify early warning signs of potential issues, allowing maintenance to be planned proactively.
Here are some common condition monitoring techniques used in predictive maintenance:
-
Vibration monitoring: This technique detects imbalances, misalignments, and other mechanical issues by measuring the vibrations of rotating equipment like motors and pumps. Sudden changes in vibration patterns can indicate problems before they become critical.
-
Thermography: Using infrared cameras, thermography monitors the temperature of equipment. Hot spots can reveal issues such as electrical faults or mechanical wear, which can lead to failure if not addressed.
-
Ultrasound analysis: Ultrasound sensors detect high-frequency sounds that indicate friction or leaks in systems like compressed air, hydraulic lines, or steam traps. These sounds are often inaudible to the human ear but can signal early signs of wear or malfunction.
-
Oil analysis: By examining lubricants, oil analysis can reveal the presence of contaminants, metal particles, or chemical breakdown, which can indicate internal wear or failure in engines, gearboxes, and hydraulic systems.
-
Motor current analysis: This technique monitors the electrical current drawn by motors. Variations in the current can point to issues like motor overloads, insulation breakdowns, or bearing failures.
These condition-monitoring methods and IoT sensors form the data-gathering foundation of predictive maintenance — feeding your predictive models with a high volume of real-time data.
Cloud computing and predictive algorithms to store and analyze the data
Once data is collected from machines through condition monitoring, it needs to be stored and analyzed to make accurate maintenance predictions. This is where cloud computing and predictive algorithms play a crucial role.
Cloud infrastructure provides the ability to store vast amounts of data securely and access it in real time. Instead of relying on local servers, cloud-based systems allow companies to scale their data storage and processing capacity as needed. This flexibility is essential for managing the large volumes of data generated by IoT sensors and condition-monitoring devices.
Predictive algorithms, powered by machine learning and artificial intelligence (AI), process this data to identify patterns and trends that indicate when a machine is likely to fail. Machine learning models are trained to recognize signs of wear, stress, or performance decline based on historical data and real-time inputs. As the system gathers more data, the algorithms become better at accurately predicting maintenance needs.
Incorporating these technologies into your maintenance strategy leads to fewer unexpected breakdowns and more targeted maintenance actions.
Maintenance software to trigger and execute maintenance work
After data is analyzed and maintenance needs are predicted, the next step is to trigger and execute the maintenance tasks efficiently. This is where maintenance software comes into play, often in the form of a CMMS or a smart manufacturing platform like L2L.
How does this work in a production setting? Well, if the maintenance platform you’re using supports it, you can connect your predictive maintenance model with it. You can set up automations where, based on the analyzed data, the predictive maintenance model will instruct your maintenance software to send alerts or even create and schedule work orders.
Whether using a CMMS or a connected workforce platform like L2L, maintenance software plays a critical role in executing predictive maintenance strategies timely and effectively, ensuring the right actions are taken at the right time.
An example of predictive maintenance in manufacturing
To illustrate how predictive maintenance works in practice, let’s look at a manufacturing plant where a critical piece of rotating machinery — such as a centrifugal pump — is identified as one of the assets that should be put on a predictive maintenance program.
This pump plays a key role in the production process, and any failure would result in costly downtime and delays.
In this case, we’ve implemented a combination of vibration analysis and ultrasound monitoring to track the pump’s condition in real time. Vibration sensors continuously monitor for imbalances, misalignment, or excessive friction in the rotating components, while ultrasound sensors detect any abnormal noises that may indicate the early stages of wear, such as bearing failure or leaks.
The predictive maintenance system works by collecting this data, analyzing it through machine learning algorithms, and detecting subtle changes that suggest the pump may fail soon.
For example, the system might pick up on increasing vibration levels or ultrasonic signals that indicate the early signs of bearing degradation. Based on this data, the system automatically triggers a maintenance work order before the pump fails, allowing the maintenance team to replace the bearings and avoid an unexpected breakdown.
This approach not only prevents the pump from failing but also helps optimize the maintenance schedule, reducing both unnecessary repairs and unplanned downtime.
The steps for implementing a predictive maintenance program at your plant
Implementing a predictive maintenance strategy can seem complex. To facilitate a smooth transition, let’s break it down into manageable steps:
-
Identify critical assets: Prioritize machinery or equipment that are critical to production or have high maintenance costs. Focus on equipment with a history of frequent breakdowns or high repair costs.
-
Collect equipment data: Gather historical maintenance data and start tracking real-time sensor data (e.g., vibration, temperature) from critical equipment. Utilize ERP or CMMS system data if available.
-
Choose the right sensors and IoT devices: Select sensors or IoT devices compatible with your equipment to monitor relevant parameters, ensuring they can communicate with your network or cloud infrastructure.
-
Establish data connectivity: Set up a network infrastructure to enable real-time data collection and transmission from the equipment to a centralized system. Consider using cloud-based platforms for scalable data storage and analysis.
-
Train predictive models: Use machine learning and appropriate statistical models (such as regression models, classification algorithms, or anomaly detection). Train your algorithms using historical and real-time data to predict equipment failures and maintenance needs. Validate and fine-tune models for better accuracy.
-
Set maintenance workflows: Integrate predictive insights into your existing maintenance management system. Define workflows for maintenance teams to respond to alerts, including automated work order creation.
-
Pilot the system: Implement the predictive maintenance solution on a small scale (pilot phase) to test its effectiveness. Monitor results like reduced downtime or improved performance, and make adjustments as needed.
-
Train staff: Educate maintenance and operations teams on using predictive maintenance tools, dashboards, and workflows. Ensure they understand how to interpret predictive insights and respond accordingly.
-
Scale the solution: Once the pilot is successful, expand the predictive maintenance system to cover more assets. Continuously refine models and adjust workflows as necessary based on data feedback.
By following these steps, you can successfully implement a predictive maintenance program at your plant. Your maintenance software provider should be able to help you out with the transition.
While this can take some time to get fully going, the long-term benefits — improved asset reliability, reduced downtime, and cost savings — make it a worthwhile investment.
Optimize maintenance with L2L’s predictive maintenance solution
L2L’s platform offers a comprehensive set of tools designed to help manufacturers implement and run an efficient predictive maintenance program. Unlike traditional CMMS systems, L2L’s connected workforce solution goes beyond simply tracking work orders. It integrates real-time data from your equipment, giving maintenance teams and operators immediate insights into the health of their assets.
With L2L, manufacturers can:
-
Collect and analyze real-time data: L2L connects to various sensors and IoT devices, continuously gathering data on critical assets. This data is analyzed using machine learning algorithms to identify trends and predict potential failures before they happen.
-
Automate maintenance triggers: The platform automatically generates alerts or work orders when the system detects a potential issue. This ensures that no maintenance task is missed and that teams are only working on assets that truly need attention.
-
Streamline communication and collaboration: L2L’s connected workforce solution enables real-time collaboration between maintenance, production, and other departments.
-
Improve asset visibility and performance tracking: We offer powerful reporting tools that allow manufacturers to track asset performance, maintenance history, and the impact of predictive maintenance on overall productivity.
-
Adapt and scale: L2L’s cloud-based infrastructure allows easy scaling, ensuring your PdM program grows as your business does.
See for yourself how L2L can help you run predictive maintenance at your plant when you book a demo or reach out to sales@l2l.com!
Revisions
Original version: 30 September 2024
Written by: Evelyn DuJack
Reviewed by: Daan Assen
Please read our editorial process for more information
Related Posts
Subscribe to Our Blog
We won't spam you, we promise. Only informative stuff about manufacturing, that's all.