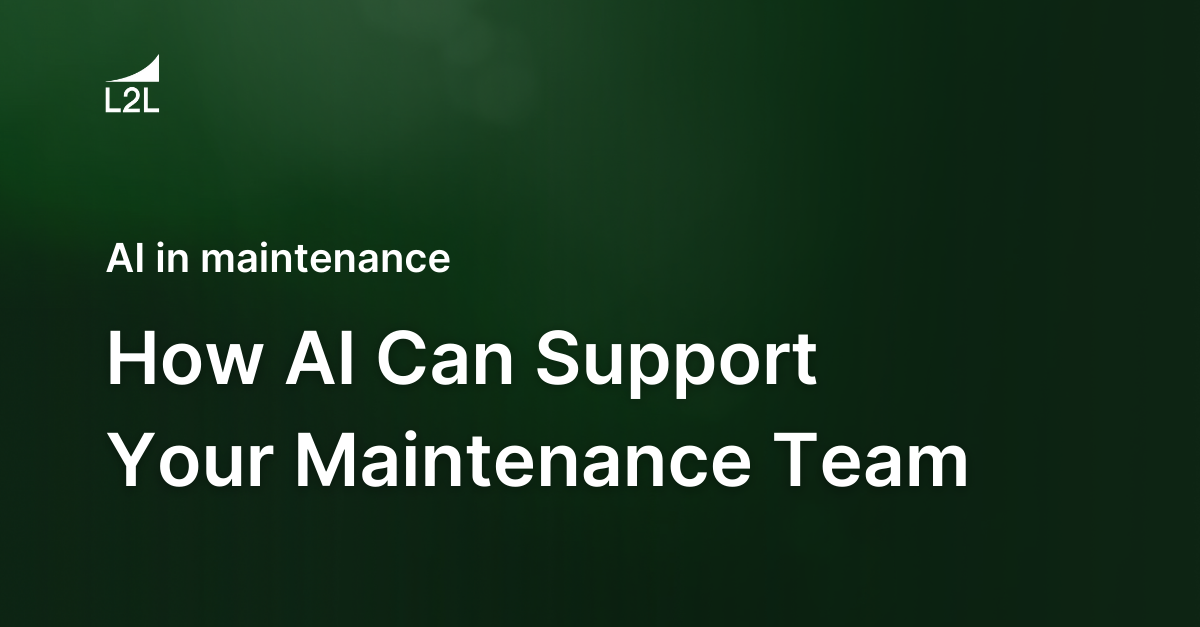
When a machine breaks down, you lose more than the time it takes to get it back online. You also lose money, productivity, and even materials in some cases.
The bad news is that breakdowns are an inevitable part of manufacturing. No matter how advanced, every factory deals with unplanned downtime.
But here’s the good news: You can dramatically reduce unplanned downtime by using artificial intelligence (AI) in maintenance—by preventing breakdowns in the first place.
In short, AI is a category of technology that mimics human intelligence, where computers perform cognitive tasks like learning, problem-solving, and content creation.
Using AI in maintenance management offers incredible benefits for manufacturers, including:
-
More efficient maintenance processes
-
Lower maintenance costs
-
Improved safety
-
Data-driven decision-making
In this article, we’ll go over AI’s role in predictive maintenance, other applications of AI in maintenance, and how to incorporate intelligent technology into your maintenance strategy.
The role of AI in predictive maintenance
One of the primary use cases of AI in manufacturing is predictive maintenance. Predictive maintenance (PdM) is a process that uses predictive analytics and Internet of Things (IoT) sensors to predict when a mechanical failure will occur. Gathering data from equipment sensors, operator inputs, and machine history, PdM systems use advanced algorithms to estimate the best times for maintenance—usually right before a machine is about to fail.
But how does AI enable these types of predictions?
AI drives predictive maintenance by:
-
Gleaning data from manufacturing assets. By collecting current and historical data from machines, production systems, and other relevant sources, PdM systems can establish a baseline for normal operation.
-
Using machine learning (ML) algorithms to parse meaningful data from the rest. PdM algorithms use predefined rules and instructions to analyze and organize datasets.
-
Continuously learning from your manufacturing system. ML algorithms take data analysis and synthesis a step further by adapting to new information. This iterative process lets PdM systems “learn” about your maintenance needs and respond to them with more precision as time goes on.
AI enables predictive maintenance systems to compare current and baseline machine data, alerting maintenance teams to anomalies long before they result in costly breakdowns. This translates to substantial cost savings and productivity gains in the long term.
Other applications of AI in maintenance management
AI’s use in equipment maintenance extends beyond standard PdM use cases. In fact, many industrial companies use AI to enhance a wide range of maintenance and maintenance-adjacent processes.
Here are some other major ways AI can be a valuable tool in maintenance management.
Automated maintenance scheduling
AI can analyze data from machines, historical maintenance data, and operational systems to identify optimal maintenance schedules for each type of equipment. It can automate maintenance scheduling based on machine conditions and further tailor those schedules based on factors like procurement, production schedules, and workforce availability.
For example, an AI-driven system can continuously monitor vibration and temperature in production machines and identify subtle signs of wear or potential breakdowns. When the AI detects a potential issue, it automatically schedules a maintenance task during the least disruptive production window, notifies the maintenance team, and ensures that any necessary parts are pre-ordered. This proactive approach minimizes unplanned downtime and keeps production lines running smoothly
Fault detection and diagnosis
AI can help detect and diagnose mechanical problems by collecting and analyzing sensor data from equipment and machinery. This process, known as condition monitoring, uses techniques like thermography and ultrasound analysis to identify abnormalities. From there, predictive algorithms analyze the data for signs of specific issues like machine damage or inefficiencies.
For example, if machine sensors detect abnormal vibrations in an extruder machine, they immediately send that data to a PdM system for analysis. The system then alerts the maintenance team to assess the issue. Once the team confirms the problem—a loose front plate bolt—they can stop the machine to tighten the bolt before it causes serious damage.
Supply chain optimization
Using AI in spare parts inventory management and procurement can improve supply chain resilience and prevent issues like stockouts or overstocking. Managing maintenance activities becomes easier when you stabilize the flow of goods through your facility. This is especially true if you use predictive analytics to align production capacity with demand and material availability.
For instance, your PdM system can help you determine precisely when a conveyor will need a new adapter shaft based on its maintenance history and current condition. If your demand forecasting system detects potential disruptions with your regular supplier, you may decide to stock up on extra parts to mitigate future shipping delays.
Natural language processing (NLP) for maintenance requests
You can also use AI to streamline maintenance requests. Natural language processing (NLP) technologies can analyze notes made by operators and technicians to determine the correct spare parts for a maintenance request and even diagnose issues.
One example of NLP in maintenance is an AI-powered chat feature embedded in modern connected worker platforms. If an operator is notified of a mechanical problem, they may pull up a chat window to ask an AI assistant for troubleshooting assistance. If the problem persists, the system will automatically notify the maintenance team of the issue.
Another example is NLP-enabled software that can “read” submitted work requests. When an operator logs a maintenance issue, the NLP system scans the request, extracts essential details, and automatically generates a draft work order. This draft is sent to a maintenance planner or manager, who can approve, edit, or dismiss the order, reducing administrative work.
Remote assistance and monitoring
AI plays a key role in enhancing manufacturing maintenance by enabling remote assistance and monitoring through advanced technologies like augmented reality (AR) and virtual assistants. These AI-driven tools allow technicians to troubleshoot equipment issues from anywhere, using real-time data and visual guides to identify and resolve problems quickly. This improves efficiency and cuts costs by reducing downtime and the need for on-site visits.
For example, AR-powered maintenance tools can overlay digital instructions on physical machinery, guiding operators through complex repairs. Additionally, AI virtual assistants can monitor equipment performance in real-time, predicting potential failures and providing recommendations to prevent breakdowns.
How L2L utilizes AI to simplify work for maintenance and production teams
Reaping the benefits of artificial intelligence in your maintenance strategy is easy with the help of a connected workforce platform like L2L. Our solution uses AI to put critical data at your frontline teams’ fingertips, allowing them to solve problems faster.
Maintenance and production teams can use L2L’s AI-driven capabilities to:
-
Improve performance using proactive, AI-generated recommendations based on analysis of past performance and current conditions.
-
Boost operational availability by anticipating machine issues and providing workers with the insights they need to solve problems quickly.
-
Reduce scrap using AI to analyze existing quality metrics and data so you can pinpoint quality trends and drive continuous improvement.
-
Manage inventory levels for spare parts with AI-driven usage, consumption, and scheduled PM analysis, ensuring the right parts are always available.
L2L Assist provides data-driven guidance for maintenance teams on an intuitive interface.
Ready to transform maintenance processes in your facility?
Get started with L2L Assist or reach out to our manufacturing intelligence experts at sales@l2l.com.
Revisions
Original version: 25 October 2024
Written by: Evelyn DuJack
Reviewed by: Daan Assen
Please read our editorial process for more information
Subscribe to Our Blog
We won't spam you, we promise. Only informative stuff about manufacturing, that's all.